Enhancing Business Efficiency through Spam Filter Machine Learning
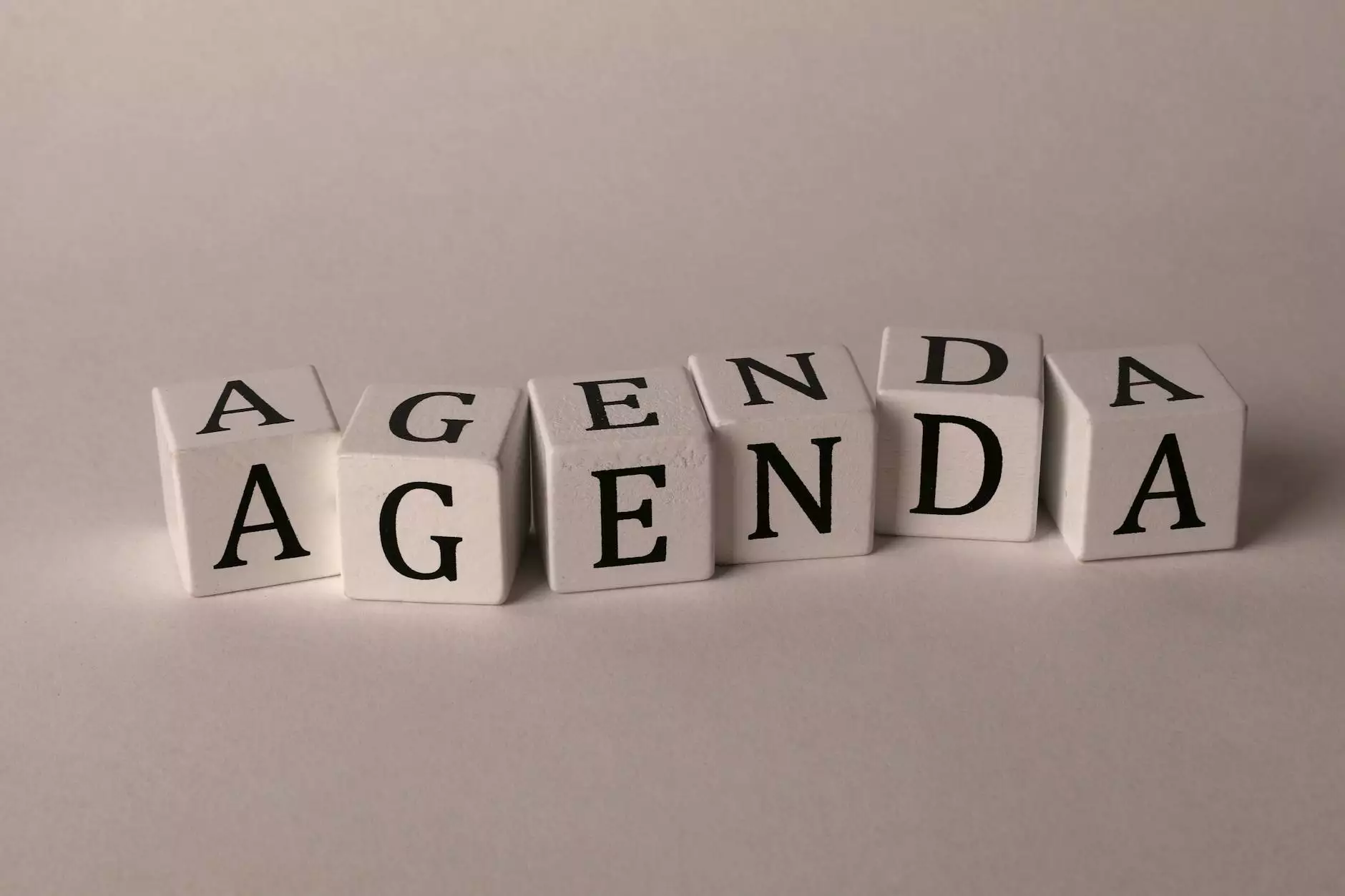
In today's digital landscape, where data flows incessantly, businesses face the critical challenge of managing vast quantities of email communication. Spam emails not only clutter inboxes but also pose significant security threats. For companies seeking to optimize their operations, incorporating spam filter machine learning into their IT and security systems is essential.
Understanding Spam Filter Machine Learning
Spam filter machine learning is an advanced technology that utilizes algorithms to identify and filter out unwanted email messages. Unlike traditional spam filters that rely on predefined rules, machine learning models learn from data, improving their accuracy over time. This adaptability makes them particularly efficient in combating sophisticated spam tactics.
Why is Spam Filter Machine Learning Essential for Businesses?
Businesses can gain several advantages from implementing spam filter machine learning:
- Improved Email Management: By significantly reducing the volume of spam, businesses can ensure that employees spend more time on productive tasks rather than sorting through irrelevant emails.
- Enhanced Security: Spam filters using machine learning can detect phishing attempts and malware-laden emails, protecting sensitive corporate data.
- Cost Efficiency: Automating spam filtering saves businesses time and resources that can be allocated to other critical areas.
- Scalability: As a business grows, the volume of emails increases. Machine learning models can scale accordingly to maintain high levels of filtering accuracy.
How Spam Filter Machine Learning Works
The mechanism behind spam filter machine learning involves several key processes:
1. Data Collection
The first step in training a machine learning model is gathering data. This includes:
- Email Features: Subject lines, sender addresses, and message content.
- Labeling: Emails are categorized as either "spam" or "not spam" based on historical data.
2. Feature Extraction
Once data is collected, the next step is to identify relevant features that the model will analyze. This might include:
- Frequency of certain keywords.
- Email metadata such as time and sender patterns.
- Structural elements like hyperlinks and attachments.
3. Model Training
The selected features are then used to train the machine learning model. Common algorithms used in spam filtering include:
- Naive Bayes: A statistical method based on applying Bayes' theorem with strong independence assumptions.
- Support Vector Machines (SVM): A supervised learning model integrating classification and regression tasks.
- Ensemble Methods: Techniques that combine multiple models to improve prediction accuracy.
4. Continuous Learning and Improvement
One of the most powerful aspects of spam filter machine learning is its ability to learn continuously. Feedback loops allow the system to adapt to new spam tactics, improving detection rates over time.
The Benefits of Machine Learning in Spam Filtering
Integrating spam filter machine learning into IT services and computer repair processes has several distinct advantages:
Accuracy
Machine learning models can analyze vast datasets and identify patterns that might go unnoticed by human operators. This results in a significantly lower rate of false positives, meaning valid emails are less likely to be misclassified as spam.
Speed
The real-time processing capabilities of machine-learning algorithms ensure that spam is identified and filtered swiftly, providing immediate protection to users without delay.
Adaptability
As spammers continuously evolve their tactics, machine learning models can adjust and retrain with newer data, maintaining their effectiveness against emerging threats.
User Empowerment
With an effective spam filtering system in place, employees can focus on their core responsibilities instead of wasting time dealing with spam. This leads to increased productivity and job satisfaction.
Implementing Spam Filter Machine Learning in Your Business
For businesses aiming to adopt spam filter machine learning, here are some actionable steps:
1. Assess Your Current Email Infrastructure
Begin by evaluating your existing email systems and identifying how well they currently manage spam. This assessment can help pinpoint weaknesses that the new solution should address.
2. Choose the Right Technology Partner
Select a software provider or IT service company with expertise in machine learning algorithms for spam filtering. Consider their track record of innovation and user feedback.
3. Train Your Staff
Educate employees on recognizing spam emails and the importance of reporting misclassified messages. This feedback can be invaluable in enhancing the system's learning process.
4. Monitor and Optimize
After implementation, it’s crucial to monitor the performance of your spam filtering solution. Regularly review spam detection rates, false positive incidents, and overall effectiveness to make necessary adjustments.
Real-World Success Stories
Many organizations have successfully integrated spam filter machine learning into their operations, resulting in notable improvements:
Case Study 1: Tech Firm
A prominent tech company adopted a machine learning-based spam filter and reported a 40% reduction in spam-related incidents within the first three months. This allowed their IT department to allocate resources towards other strategic initiatives.
Case Study 2: Financial Institution
A reputed bank utilized spam filter machine learning to enhance their cybersecurity measures. They achieved a 50% decrease in phishing attempts, thereby safeguarding customer data and preserving trust in their services.
Challenges and Considerations
While the benefits are significant, businesses should also be mindful of potential challenges:
- Complexity: Implementing machine learning solutions can be challenging; it requires skilled personnel and technical know-how.
- Initial Costs: The upfront investment in technology and training may be substantial, but the long-term savings can justify these expenses.
- Data Privacy Concerns: Businesses must ensure they handle email data responsibly to comply with regulations such as GDPR.
The Future of Spam Filter Machine Learning
The evolution of spam filter machine learning is ongoing. As innovation in AI and machine learning continue to advance, businesses can expect even more improved resilience against spam threats. Future trends may include:
- Integration with Other AI Systems: Merging spam filtering capabilities with broader cybersecurity frameworks for enhanced protection.
- Using Natural Language Processing (NLP): To better comprehend the context and sentiment of emails, leading to smarter filtering decisions.
- Personalization: Filters that adapt to individual user preferences and habits, significantly refining email categorization.
Conclusion
In summary, the integration of spam filter machine learning is rapidly becoming a necessity for businesses aiming to enhance their operational efficiency and security. By reducing spam, safeguarding assets, and allowing employees to focus on their core tasks, businesses can reap the benefits of a more streamlined and secure communication infrastructure. As we move into a future increasingly defined by data, taking steps to adapt and improve our technological defenses will yield significant dividends in the competitive business landscape.